Big Things Ahead for AI in 2023: Predictions
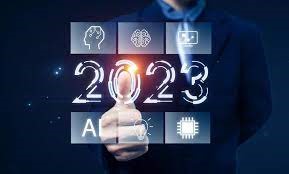
By Alex Woodie–The AI train has been gaining steam for several years now, and nothing appears ready to stop it (except for bad data, that is). With momentum building, which direction will AI head in 2023? We leave that to the experts.
Many AI projects are ill-conceived and ultimately fail for that reason. In 2023, enterprises will find a new vigilance when it comes to assessing the efficacy of AI, says Zohar Bronfman, co-founder and CEO of Pecan AI.
“In 2023, business leaders will evaluate potential data science projects much more rigorously than in the past. These projects often fail to generate real impact due to poor alignment with business needs or because they never make it into production. With the expense and time commitment involved in data science, leaders will scrutinize proposed efforts more carefully and investigate the right way to pursue them to ensure that business-improvement actions could be taken in the near term based on the output of the models — or scuttle them before resources are wasted,” Bronfman says.
Demand for data scientists will be up in 2023. So will demand for GPUs to train deep learning models, predicts Nick Elprin, the CEO and co-founder of Domino Data Lab.
“The biggest source of improvement in AI has been the deployment of deep learning–and especially transformer models–in training systems, which are meant to mimic the action of a brain’s neurons and the tasks of humans. These breakthroughs require tremendous compute power to analyze vast structured and unstructured data sets. Unlike CPUs, graphics processing units (GPUs) can support the parallel processing that deep learning workloads require. That means in 2023, as more applications founded on deep learning technology emerge to do everything from translating menus to curing disease, demand for GPUs will continue to soar,” Elprin says.
Seconding that motion is Charlie Boyle, vice president of DGX systems at Nvidia, which hopes to sell many more GPUs next year.
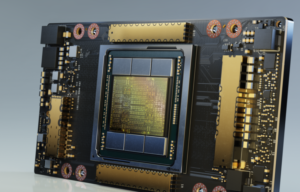
Nvidia’s A100 GPU
“In 2023, inefficient, x86-based legacy computing architectures that can’t support parallel processing will give way to accelerated computing solutions that deliver the computational performance, scale and efficiency needed to build language models, recommenders and more. Amidst economic headwinds, enterprises will seek out AI solutions that can deliver on objectives, while streamlining IT costs and boosting efficiency. New platforms that use software to integrate workflows across infrastructure will deliver computing performance breakthroughs –with lower total cost of ownership, reduced carbon footprint and faster return on investment on transformative AI projects–displacing more wasteful, older architectures.”
You’re about as likely to hire a qualified data scientist at a reasonable rate as you are to discover a unicorn (we jest). Could 2023 be the year the world reaches “peak data scientist”? Ryan Welsh, founder and CEO of Kyndi, argues that it is.
“The shortfall of data scientists and machine learning engineers has always been a bottleneck in companies realizing value from AI. Two things have happened as result: (1) more people have pursued data science degrees and accreditation, increasing the number of data scientists; and (2) vendors have come up with novel ways to minimize the involvement of data scientists in the AI production roll out. The coincident interference of these two waves yields ‘peak data scientist,’ because with the advent of foundational models, companies can build their own applications on top of these models rather than requiring every company to train their own models from scratch. Less bespoke model training requires fewer data scientists and MLEs at the same time that more are graduating. In 2023, expect the market to react accordingly resulting in data science oversaturation,” Welsh says.
Expect to see ethical AI to continue to attract attention and resources in the enterprise, predicts Triveni Gandhi, the responsible AI lead at data science tool provider Dataiku.
“While we’ve seen headlines in the news about some companies cutting ethical AI roles, the reality is that most companies will continue investing in their ethical AI teams. This resource is crucial for the scale and operation of AI, helping companies to be confident that their AI outputs are aligned with their values and executed in a robust and reliable way. What’s more, ethical AI teams give confidence to users that the products they are interacting with are considered and meet expectations around safety and trust. For any company to stay ahead of the curve, building and enabling an ethical AI group is a must,” Gandhi says.
One of deep learning’s dilemmas is the black box nature of predictive models. One way to address that concern is to pair AI with causal knowledge graphs in 2023, says Jans Aasman, the CEO of graph database maker Franz.
“The next few years will see growth in Causal AI starting with the creation of Knowledge Graphs that discover causal relationships between events. Healthcare, pharma, financial services, manufacturing and supply chain organizations will link domain-specific knowledge graphs with causal graphs and conduct simulations to go beyond correlation-based machine learning that relies on historical data. Causal predictions have the potential to improve the explainability of AI by making cause-and-effect relationships transparent,” Aasman says.
You won’t find much pushback on that from Maya Natarajan, the senior director of product marketing at graph database maker Neo4j, who also foresees visible progress at the junction of graph and AI.
“Enterprises will continue searching for the best ways to take advantage of knowledge graphs for responsible AI. By leveraging the context knowledge graphs provide, organizations can strengthen accuracy for ethical decision making, enhance explainability by maintaining provenance of data flows, and help mitigate biases by opening up new analysis methods,” Natarajan says.
Another type of database finding newfound traction in the Year of AI is the vector database. Or so says Edo Liberty, the founder and CEO of Pinecone, one of the early leaders in the vector database market.
“As AI continues to grow and become more widely used, there will be a corresponding need for more advanced and scalable infrastructure to support its development and deployment. One key area of investment in AI infrastructure will be in specialized data infrastructure, such as vector databases, which are designed to store and work with the large volumes of data generated by modern ML models. This will accelerate the development and deployment of AI systems that outperform even last year’s applications in a wide range of areas,” Liberty says.
Companies have been growing their use of AI in recent years, with mixed success. But in 2023, AI will enter the “less is more” phase of growth, predicts Kimberly Nevala, an advisory business solutions manager at SAS.
“AI will proliferate as organizations realize less is more and quietly shift focus away from wholesale innovation as an objective. Rather, AI will be applied to a broader spectrum of smaller decision points and actions whose collective impact is greater than the sum of the parts. Thus, paradoxically priming the pump for increasingly bold transformation as organizations and, most importantly, their employees become broadly aware and comfortable using these technologies,” Nevala says.
So you’ve invested deeply in GPUs to train your neural network. Great! But what do you do with that rocket sled when it’s not training your AI model? Well, there’s always SQL queries that could use some additional horsepower, according to Matan Libis, vice president of product at SQream.
“The ability to re-use or re-purpose computing resources for AI/ML is both an exciting and valuable opportunity we are seeing develop for enterprises. Not only does the re-purposing reduce the carbon footprint AI heavy industries are leaving behind, but a general increase in cheaper global data storage solutions lessens the need to rely on GPU hardware for other use cases. Additionally, companies can lower latency when you don’t need to move data from place to place; whereas once enterprises were preparing data in one place, training in another and shifting inference in another, the hope is that by streamlining the process we will see a huge improvement in both accuracy and speed of AI/ML capabilities,” Libis says.
The high cost of cloud computing is weighing on everybody, but AI users can fight the cost-creep by optimizing their models, says Yonatan Geifman, CEO and Co-Founder of deep learning company Deci.
“Businesses that have been running AI models in cloud environments are seeing the financial toll high-powered cloud processing can have on their bottom line. In 2023, we are likely to see more companies seeking to reduce those AI inference cloud costs. One of the most effective ways to do this is by increasing the speed of AI models while preserving their accuracy. Companies will then require less processing time on the cloud and effectively save money.”
In 2023, we’ll see more breakthroughs in self-supervised machine learning techniques that don’t require labeled data, predicts Yossi Synett, chief scientist at Evinced.
“One thing that has held back AI is a lack of high-quality labeled data. While we are already seeing progress today, growth will continue in 2023. More and more we are finding ways to pre-train models using self-supervised learning followed by fine-tuning models to a specific task. The best and most proven example of this is in NLP (natural language processing) where techniques called Masked Language Modeling (making the model predict hidden words in sentences) and Causal Language Modeling (making the model predict the next word in sentences) have totally changed the game. Since self-supervised learning doesn’t require labeled data and fine-tuning requires far less labeled data this makes it much easier to train complex models. Complementing this are new techniques that can be used to better select examples for labeling which further reduces the financial roadblock to utilizing AI,” Synett says.
Be ready for AI to reach a higher plane in 2023, with new user interaction modalities and greater understanding of intent, says Chintan Mehta, an EVP and group CIO at Wells Fargo.
“In 2023 and beyond, there will be an exponential acceleration in AI deployment and signal sensing. AI will overpower bias sensing, heuristics for judgment and legal interpretations. The industry will build more solutions for bias breaking so AI can provide the consumer with the solution while explaining its course of action. User interfaces will transition. They’ll move beyond app-based experiences that stem from non-visual tap touch interactions to transferring to context visual calls to action as well as language and gesture based interactions. The AI needed to power these experiences will increase aggressively, advancing beyond understanding just the language to truly master the hidden intent of each interaction. AI will generate AI.”
In 2023, we’ll see never-before-possible AI and machine learning use cases emerge and ultimately become mainstream, predicts Marco Santos, the CEO of USA & Latin American Regino for German IT firm GFT.
“As companies break free from the constraints of legacy systems and are able to bring together massive data sets from disparate systems, we’ll see a slew of never-before-possible use cases for AI and machine learning. In auto manufacturing, for instance, we’re just starting to see the emergence of next generation manufacturing data platforms, or single unified cloud-based platforms where manufacturers are aggregating all data across their entire organizations. Once the data’s in there, they can start building AI-enabled applications against that.”
Category: Uncategorized